Back in August of 2024, Navicat released version 17.1, which added Enhanced Query Explain and Expanded Database Connectivity. Now, version 17.2 is in Beta and is slated for release shortly. Some of the new features that we'll be talking about in today's blog include:
In today's microservices-driven world, organizations face increasing challenges in managing data across distributed systems. Database Mesh Architecture has emerged as a powerful solution to these challenges, offering a decentralized approach to data management that aligns with modern application architectures. This article explores how Database Mesh Architecture works and how to implement it using popular databases such as PostgreSQL and MongoDB.
The landscape of data storage and management is currently undergoing a dramatic transformation. As organizations deal with increasingly diverse types of data, traditional relational databases are no longer sufficient for many modern applications. Enter multi-modal databases, a powerful solution that's reshaping how we think about data storage and manipulation. This article explores how multi-modal databases are revolutionizing data management by enabling organizations to store and process multiple types of data - from traditional tables to documents, graphs, and vectors - all within a single, unified system.
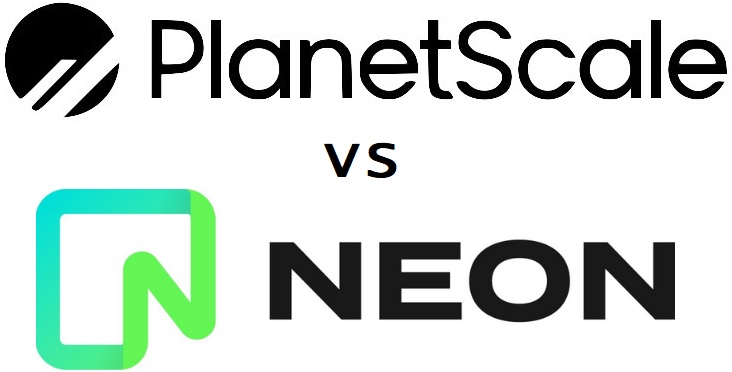
According to the 2023 Stack Overflow Developer Survey, PostgreSQL has achieved a significant milestone by overtaking MySQL as the most admired and desired database system among developers. This shift reflects a growing appreciation for PostgreSQL's robust feature set, reliability, and extensibility in the developer community.
This changing landscape has sparked innovation in the database-as-a-service space, particularly evident in the competition between two cutting-edge platforms: PlanetScale, built on MySQL, and Neon, powered by PostgreSQL. Both services are reimagining how developers interact with databases in the cloud era. These developments should be of interest to Navicat users, as both are fully supported by Navicat's comprehensive database development and management tools.
This blog will provide a comparison of the two services and offer some tips for choosing between them.
Range Types
It's no secret that PostgreSQL is one of the most flexible databases on the market. In fact, PostgreSQL's extensibility and rich feature set recently propelled PostgreSQL ahead of MySQL as the most admired and desired database system among developers. In this series on creating custom data types in PostgreSQL using Navicat Premium 17 we've explored a few options so far, including custom Domains, as well as Composite and Enumerated types. The topic of this week's blog will be Range types, which are particularly useful when you need to work with continuous intervals or ranges of values.
- 2025 (1)
- 2024 (1)
- 2023 (1)
- 2022 (1)
- 2021 (1)
- 2020 (1)
- 2019 (1)
- 2018 (1)
- 2017 (1)